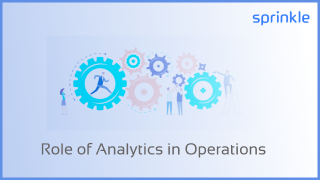
Efficient operations management is crucial in today's rapidly changing business environment to ensure sustainable growth and competitive advantage. The world of operations management has transformed due to the power of analytics uncovering and conveying meaningful patterns generated from actionable user data.
In this article, we will discuss the role of data analytics in operations management, its benefits, some key components of operations management, real-world use cases, and the challenges faced in the process of operational analytics.
What is Operational Analytics and Types of Analytics Used in Operations Management?
Operational analytics refers to the process of using data and advanced analytical techniques to improve and optimize day-to-day operations within an organization. It involves collecting, analyzing, and interpreting real-time data from various sources.
Organizations use operational analytics to uncover trends and valuable insights from real-time data. There are various types of business analytics commonly used in operations management, each serving a different purpose.
The main types of analytics: are descriptive, predictive, and prescriptive.

Descriptive Analytics:
Descriptive analytics is the fundamental form of analytics that focuses on summarizing historical data to understand what happened in the past. It provides an overview of the current scenario and allows businesses to identify trends, patterns, and relationships within their business operations. This type of analysis answers questions like "What happened?"
Descriptive analytics can be used to monitor key performance indicators (KPIs) such as output, inventory levels, or customer satisfaction scores.
Predictive Analytics:
Predictive analytics takes descriptive analysis one step further by using historical data to make predictions about future outcomes. It utilizes statistical models and machine learning algorithms to predict possible scenarios based on existing patterns. This type of analysis answers questions like "What can happen next?"
Predictive analytics enables businesses to foresee future demand, optimize resource allocation, and improve decision-making processes.
Prescriptive Analytics:
Prescriptive analytics goes beyond just predicting the future as it provides recommendations on how to optimize decisions and actions based on predicted outcomes. It answers questions like "What should we do now?"
Prescriptive analytics helps businesses identify the most efficient production schedules, supply chain routes, or inventory levels.
Uses of Analytics in Operations Management Process
Analytics in operations management helps in revealing hidden patterns and trends within large volumes of operational data. Some of the uses of analytics in operations management are listed below:
Past sales analysis using customer data can be analyzed to notice deviations in customer preferences that help businesses predict future, demand accurately while modifying production levels to avoid stock shortages or surplus inventory thus helping in customer relationship management.
Operational analytics can optimize businesses' supply chains by providing up-to-date information on supplier competence and inventory levels. This allows organizations to make clear choices, ensuring that they acquire sufficient materials at the right moment.
Predictive maintenance is another important use of analytics in operations management whereby organizations can proactively detect early signs of malfunction or failure by examining sensor data from machines or devices.
Leverage operational analytics as it is useful in improving workforce planning and scheduling. Through an assessment of past employee performance records alongside external factors like customer demand patterns, establishments of staffing levels can be done to ensure adequate coverage while downsizing labor expenses.
Key Components of Operations Management
Operations management is a crucial aspect of any business that involves the design, implementation, and control of processes used to transform inputs into desired outputs. It contains various key components such as process design, capacity planning, and inventory management. Let's explore each of these components in detail.
Process Design:

Process design refers to the creation or modification of processes involved in producing goods or delivering services. It aims to optimize efficiency, quality, and customer satisfaction while minimizing costs.
The process design involves:
- determining the series of actions and activities,
- recognizing resources that are required,
- defining work procedures, and
- establishing key performance metrics
Capacity Planning:

Capacity planning involves determining the optimal level of constructive resources needed to meet current and future demand effectively. It ensures that an organization has sufficient capacity to fulfill customer orders without delays or excessive costs.
Capacity planning considers factors like:
- Market forecasts according to ongoing trends,
- Manufacturing capabilities of goods,
- Equipment availability,
- Labor availability, and
- Technological Advancements.
Inventory Management:

Inventory management focuses on managing the flow of raw materials, work-in-progress, and finished goods within an organization. An efficient inventory management system strives to maintain adequate stock levels while minimizing carrying costs associated with excess inventory.
Key aspects of inventory management include:
- Accurate forecasting of demand patterns,
- Timely procurement of raw materials/components,
- Monitoring product expiration dates, and
- Efficiently managing returns/defects.
Real-World Use Cases of Analytics in Operations Management
Predictive Maintenance at United Airlines:

United Airlines uses predictive analytics algorithms to optimize aircraft maintenance plans and reduce downtime. By analyzing historical maintenance records, flight data, and sensor readings from their airplanes, they can identify possible issues before they happen.
This approach allows them to plan maintenance activities efficiently, minimize delays due to unexpected breakdowns, and ultimately improve customer experience by reducing flight cancellations.
Inventory Optimization at Walmart:

Walmart is well-known for its efficient supply chain management system, which depends heavily on data-driven inventory optimization techniques. By leveraging past sales data and external factors, Walmart uses predictive models to accurately forecast market needs for each product at every store location.
This allows them to maintain optimal stock levels, avoid overstocking or understocking situations, reduce storage costs, and ensure products are readily available for customers.
Demand Forecasting at Amazon:

As one of the largest online retailers globally, Amazon relies on sophisticated analytics techniques for accurate demand forecasting. By collecting data on customer behavior patterns such as browsing history, purchase habits, and product reviews, Amazon can predict future demand with great precision.
This allows them to optimize inventory levels, minimize out-of-stock situations, and offer faster delivery times.
Challenges Along With their Solutions Faced in Operations Management Process
In today's data-driven business environment, analytics has become an essential tool for operations management. Like any other technology in the space, including analytics in operations management, comes with some challenges. In this section, we will explore some common hindrances faced by organizations and discuss strategies to overcome them while maximizing the benefits of analytics.
1. Data Integration and Data Management
One of the primary challenges in implementing analytics in operations management is ensuring the quality and integration of data. Businesses have vast amounts of data across multiple systems and platforms either a data warehouse or a data lake. Inconsistent formats, incomplete records, and lack of standardization can hamper the accuracy and reliability of insights derived from analytics.
Resolution: To overcome this challenge, organizations must invest in data integration and management platforms as these platforms ease the tedious process by setting clear guidelines for data mining, collection, storage, cleansing, and integration. Utilizing a data warehouse can streamline data flow, ensuring that accurate information is available for analysis.
2. Skill Gap Between Employees
Another significant challenge faced by organizations is a skill gap among employees. Understanding statistical concepts, programming languages like R or Python, and understanding of analytical tools can be significantly tough for those without a technical background.
Resolution: Offering training programs or workshops on basic statistical concepts and analytics tools can bridge the skill gap significantly.
3. Privacy and Security Concerns
With increased reliance on data, there is an inherent risk of privacy breaches or security threats. Protecting sensitive information while still using analytics poses a significant challenge for many organizations.
Resolution: Implement robust data protection measures to address privacy concerns. This includes encryption, access controls, regular audits, and compliance with relevant regulations.
4. Cost Concerns:
Implementing robust analytical systems and hiring skilled professionals can be expensive. Organizations need to carefully evaluate the return on investment (ROI) of these initiatives to ensure they are cost-effective.
Resolution: Prioritizing projects based on their possible impact, and conducting research before full-scale implementation, can help organizations manage costs, or investing in a low code analytics tool can help business users save costs.
Conclusion
Incorporating analytics into operations management offers tremendous potential for businesses to optimize processes, thus using operational analytics platforms can be beneficial. Sprinkle Data is a low-code business intelligence tool that has a user-friendly interface that allows users to easily gather, analyze, and visualize data from various sources. With its ability to handle large volumes of data in real-time, organizations can quickly adapt to changing market conditions and gain a competitive edge in the market.
Frequently Asked Questions- FAQ's
Why is data important for operation management?
Data is important for operations management because by analyzing data, businesses can identify trends, patterns, and opportunities for optimization in areas such as production, inventory management, supply chain, and customer service.
What are operations management and analytics?
Operations management and analytics refer to using data analysis techniques and tools to optimize operational processes. It involves using quantitative methods to analyze data and make data-driven decisions to improve business operations.
How can data analytics improve operations?
Data analytics can improve operations by uncovering hidden insights from large volumes of data. It can help identify inefficiencies within the business process, predict demand patterns, optimize resource allocation, reduce costs, and enhance productivity.
What is the role of data operation?
The role of data operation is to ensure the availability, reliability, security, and integrity of data within an organization. This includes tasks such as data mining, collection, storage, processing, cleaning, integration, transformation, and governance.
What is data analysis in operations?
Data analysis in operations refers to examining raw data to discover meaningful patterns or insights that can inform decision-making. It involves applying various statistical techniques to derive valuable information from data sets.
What is the difference between data analytics and data operations?
Data analytics focuses on extracting insights from data through mathematical or statistical techniques to support decision-making and improve business performance. Data operations are concerned with managing the technical aspects of data infrastructure such as database administration or ETL (extract-transform-load) processes.
How is operations research related to data science?
Operations research is a field that uses mathematical modeling and optimization techniques to solve complex problems related to decision-making in operations management. Data science encompasses a broader range of disciplines including statistics, machine learning, artificial intelligence, computer science, and domain expertise.
What is the difference between data science and operations management?
The main difference between data science and operations management is their focus. Data science primarily deals with extracting insights and knowledge from data, while operations management focuses on managing and optimizing operational processes.
What is data science operations?
Data science operations refer to the processes and practices involved in deploying, monitoring, and maintaining data-driven models or systems in production. It involves tasks such as model deployment, performance monitoring, troubleshooting, and continuous improvement of data products or solutions.
What is data analytics and Operations Research?
Data analytics and Operations Research are both analytical approaches used for decision-making in business settings. However, Operations Research typically focuses on mathematical modeling and optimization techniques to solve complex problems, while data analytics encompasses a broader range of techniques including statistical analysis, machine learning, and visualization.