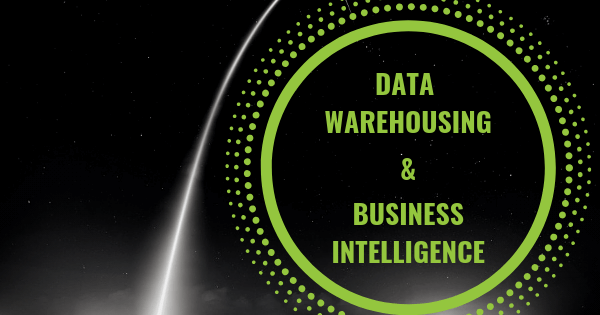
In today's digital age, businesses are accumulating vast volumes of data from various sources, ranging from customer interactions to operational metrics. This influx of data presents both challenges and opportunities. To harness the potential of this data for strategic decision-making, organizations employ sophisticated systems like data warehouses and business intelligence (BI) tools. Let's delve deeper into the intersection of business intelligence and data warehouses, exploring how they work together to extract valuable insights from complex datasets.
Understanding Data Warehousing
A data warehouse is a specialized repository that consolidates structured data from different sources into a unified format, optimized for analysis and reporting. The data warehouse plays a pivotal role as a central platform for data consolidation and analysis, particularly in structuring data for business intelligence (BI) purposes. It acts as a central storage facility where historical data from multiple systems across an organization is stored, organized, and made accessible for BI purposes.
What is a Data Warehouse?
A data warehouse is a database designed to store large volumes of historical data that can be used for analysis and reporting.
The primary function of a data warehouse is to provide a structured environment for querying and analyzing data, making it a cornerstone of modern BI architectures. Unlike transactional databases optimized for day-to-day operations, data warehouses are tailored for analytical queries that require aggregated, historical, and cross-functional data.
Role of Business Intelligence in Data Warehousing
Business intelligence encompasses technologies, applications, and processes for collecting, integrating, analyzing, and presenting business information. When integrated with data warehousing, BI empowers organizations to transform raw data into actionable insights.
Business Intelligence and Data Warehousing: A Synergistic Relationship
Business intelligence and data warehousing are closely intertwined, forming a symbiotic relationship where BI tools leverage the structured environment of data warehouses to extract, analyze, and visualize data. This synergy enables organizations to:
- Gain Insights: BI tools use data warehouses as a foundation to perform complex queries, aggregations, and calculations, unveiling trends and patterns that drive informed decision-making.
- Enable Data-Driven Decisions: By providing user-friendly interfaces and dashboards, BI tools empower stakeholders to explore data intuitively and make data-driven decisions swiftly.
- Enhance Performance Monitoring: BI tools integrated with data warehouses enable real-time or near-real-time monitoring of key performance indicators (KPIs), fostering proactive decision-making.
Business Intelligence Tools in Data Warehousing
Business Intelligence (BI) tools play a pivotal role in transforming 'the data' stored in data warehouses into actionable insights. These tools empower users to explore, analyze, and visualize 'the data' efficiently, turning raw information into meaningful business intelligence. Let’s explore the landscape of BI tools commonly integrated with data warehouses and their key features.
Types of Business Intelligence Tools
Business Intelligence tools encompass a diverse range of applications and technologies tailored for data analysis and reporting. Some prominent categories of BI tools used in conjunction with data warehouses include:
- Reporting Tools: These tools generate predefined or ad-hoc reports summarizing key performance indicators (KPIs), operational metrics, and trends. Examples include Crystal Reports, SSRS (SQL Server Reporting Services), and JasperReports.
- Data Visualization Platforms: Visualization tools convert complex datasets into intuitive charts, graphs, and dashboards, enabling users to grasp insights at a glance. Widely adopted platforms in this category include Tableau, Power BI, QlikView, and Looker.
- OLAP (Online Analytical Processing): OLAP tools facilitate multidimensional analysis, allowing users to slice and dice data along various dimensions to explore relationships and trends. Notable OLAP solutions include Oracle Essbase, IBM Cognos TM1, and Microsoft Analysis Services.
- Advanced Analytics and Data Mining Tools: These tools leverage statistical modelling, machine learning, and predictive analytics to uncover hidden patterns, correlations, and outliers within data. Examples include IBM SPSS, SAS Enterprise Miner, and RapidMiner.
- ETL (Extract, Transform, Load) Tools: While primarily used for data integration, ETL tools like Informatica and Talend also offer basic data transformation and cleansing functionalities crucial for preparing data before loading into the warehouse.
Key Features and Capabilities
Each category of BI tools offers distinct features and capabilities that enhance data analysis and decision-making within a data warehousing environment:
- Interactive Dashboards: BI tools enable the creation of interactive dashboards that provide real-time insights into KPIs and performance metrics. Users can drill down into specific data points for deeper analysis.
- Ad-Hoc Querying: Business users can create ad-hoc queries without extensive technical expertise, empowering them to explore data independently and derive actionable insights on the fly.
- Data Collaboration: Many BI tools support collaborative features that facilitate data sharing, annotations, and discussions among users, promoting a data-driven culture within organizations.
- Predictive Analytics: Advanced BI tools incorporate predictive modelling capabilities, enabling businesses to forecast trends, anticipate customer behaviour, and optimize resource allocation.
- Data Integration and Connectivity: BI platforms seamlessly integrate with data warehouses and a wide array of data sources, ensuring comprehensive data access for analysis.
- Mobile Compatibility: Modern BI tools offer mobile-friendly interfaces, allowing users to access dashboards and reports on smartphones and tablets, fostering flexibility and agility in decision-making.
Integrating BI Tools with Data Warehousing
Integrating BI tools with data warehouses involves several steps:
- Data Extraction: BI tools connect to data warehouses and extract relevant datasets for analysis. This process may involve defining data models, selecting dimensions, and specifying aggregation levels.
- Data Transformation: Before analysis, data may require transformation and cleansing to ensure consistency and accuracy. BI tools often provide built-in capabilities for data preparation.
- Data Analysis and Visualization: Once data is loaded into the BI tool, users can analyze it using a variety of visualization techniques such as charts, graphs, heatmaps, and geospatial representations.
- Report Generation and Distribution: BI tools facilitate the creation of customized reports and automate report distribution to stakeholders, ensuring timely access to insights.
Leveraging Data Mining in Data Warehousing
Data mining is a critical component of BI in data warehousing. It involves applying statistical techniques, machine learning algorithms, and artificial intelligence to identify patterns, correlations, and anomalies within large datasets. The data mining process is essential for sifting through large batches of data in a data warehouse, identifying patterns and trends, and leveraging these insights for strategic decision-making in business intelligence.
What is Data Mining?
Data mining is the process of discovering patterns and insights in large datasets using advanced analytical techniques.
In the context of data warehousing, data mining enables:
- Predictive Analytics: Anticipating future trends and outcomes based on historical data patterns.
- Segmentation and Targeting: Identifying customer segments and preferences to tailor marketing strategies.
- Anomaly Detection: Flagging unusual patterns or outliers that require further investigation.
Business Intelligence Tools for Data Warehousing
A diverse array of BI tools is available to extract value from data warehouses:
- Reporting Tools: Generate static or interactive reports summarizing key metrics.
- OLAP (Online Analytical Processing): Enables multidimensional analysis for complex queries.
- Data Visualization: Transform data into compelling charts, graphs, and dashboards for intuitive insights.
- Advanced Analytics Platforms: Incorporate machine learning and predictive modelling capabilities for deeper analysis.
FAQ Section
- What is the role of a data warehouse in the context of business intelligence? Business intelligence utilizes data warehousing as a centralized repository to extract, analyze, and visualize data for informed decision-making.
- How does a data warehouse differ from a transactional database? A data warehouse is optimized for analytical queries and stores historical data, whereas transactional databases prioritize real-time transaction processing.
- What is data mining, and how does it complement data warehousing? Data mining involves uncovering patterns and insights from large datasets, enhancing the analytical capabilities of data warehousing.
- What are some popular business intelligence tools used with data warehouses? Common BI tools include Tableau, Power BI, QlikView, and Looker, which integrate seamlessly with data warehouses.
- How can data warehouses improve decision-making? Data warehouses centralize and organize data, providing a reliable foundation for analytical insights that drive strategic decisions.
- What types of data are typically stored in a data warehouse? Data warehouses store structured, historical data from various operational systems, including sales, finance, and customer relationship management (CRM).
- Why is data warehousing important for business intelligence? Data warehousing ensures data consistency, accuracy, and accessibility, facilitating meaningful analysis and reporting.
- How does data warehousing support data-driven cultures within organizations? By providing a unified view of data across departments, data warehouses promote data-driven decision-making and collaboration.
- What are the steps involved in implementing a data warehouse? Implementing a data warehouse involves data modelling, extraction, transformation, loading (ETL), and integration with BI tools.
- What are the benefits of using OLAP (Online Analytical Processing) in data warehousing? OLAP enables multidimensional analysis, allowing users to drill down into data hierarchies and explore relationships.
- How does data warehousing contribute to regulatory compliance? Data warehouses facilitate auditability and data governance, supporting compliance with regulations like GDPR and HIPAA.
- What role does data warehousing play in data quality management? Data warehouses enforce data quality standards by consolidating data from multiple sources and resolving inconsistencies.
- How can data mining techniques be applied within a data warehouse? Data mining techniques like clustering, classification, and regression can be employed to discover hidden patterns and relationships.
- What are the scalability considerations for data warehousing? Scalable data warehouse architectures accommodate growing data volumes and user demands without sacrificing performance.
- How does business intelligence support strategic planning? BI tools facilitate scenario analysis, trend forecasting, and competitive intelligence, aiding strategic planning efforts.
- What is the significance of real-time analytics in data warehousing? Real-time analytics enable organizations to respond swiftly to changing market conditions and operational events.
- How does data warehousing promote data democratization within organizations? Data warehouses empower users across departments with self-service BI tools, reducing dependency on IT for data access.
- What are the challenges associated with data warehousing and business intelligence implementations? Challenges include data integration complexities, scalability issues, user adoption barriers, and ensuring data security.
- How does data warehousing facilitate historical trend analysis? Data warehouses retain historical data, allowing organizations to analyze long-term trends and performance metrics.
- What role does metadata management play in data warehousing? Metadata management ensures data lineage, governs data definitions and enhances data discoverability within the warehouse.
Conclusion
In conclusion, business intelligence in data warehousing represents a strategic imperative for organizations seeking to unlock the value of their data assets. By leveraging advanced analytics, data mining, and BI tools within a centralized data warehouse, businesses can gain actionable insights that drive innovation, operational efficiency, and competitive advantage in today's data-driven landscape.