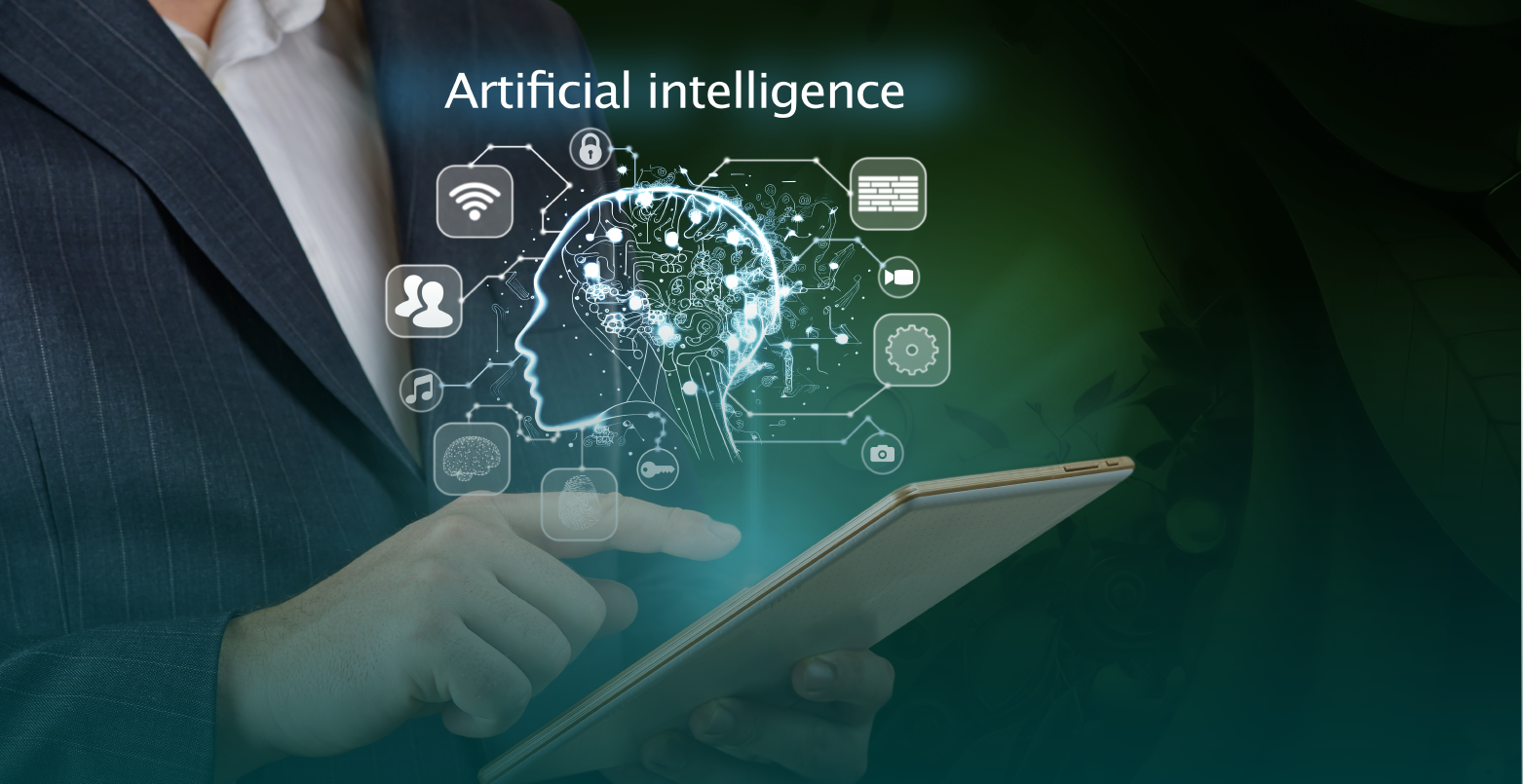
In recent years, the integration of artificial intelligence (AI) into analytics has revolutionized the way organizations derive insights from data. This transformative synergy between AI and analytics has empowered businesses to extract deeper, more actionable insights, driving efficiency, innovation, and competitive advantage across various industries. This article will delve into the profound impact of AI on analytics, exploring key concepts, applications, challenges, and future prospects.
Understanding AI and Analytics
Before delving into their intersection, it's essential to define AI and analytics:
- Artificial Intelligence (AI): AI refers to the simulation of human intelligence in machines that are programmed to think and mimic human actions. Machine learning (ML) and deep learning (DL) are subfields of AI that focus on enabling systems to learn and improve from experience without explicit programming.
- Analytics: Analytics involves the systematic computational analysis of data or statistics, aimed at discovering meaningful patterns, trends, and insights to aid decision-making.
The Evolution of AI in Analytics
The evolution of AI in analytics can be traced through several key phases:
- Descriptive Analytics: Initially, analytics primarily focused on descriptive statistics and visualization to summarize historical data and understand what happened.
- Predictive Analytics: With the advent of AI, predictive analytics emerged, leveraging statistical models and machine learning algorithms to forecast future outcomes based on historical data patterns.
- Prescriptive Analytics: AI-driven prescriptive analytics takes it a step further by suggesting optimal actions and decisions based on predictive insights, enabling proactive decision-making.
- Cognitive Analytics: The latest evolution incorporates cognitive computing, where AI systems can understand, reason, and learn from data like humans, enabling more nuanced and context-aware analysis.
Key Roles of AI in Analytics
AI brings several critical capabilities to analytics that significantly enhance its scope and impact:
- Advanced Data Processing:some text
- AI algorithms excel in handling large volumes of structured and unstructured data, enabling faster data processing and analysis.
- Natural Language Processing (NLP) facilitates text analysis, sentiment analysis, and language translation, expanding the types of data that can be analyzed.
- Enhanced Predictive Modeling:some text
- AI-powered predictive models are more accurate and adaptable, incorporating complex patterns and dependencies in data.
- Techniques like ensemble learning and deep learning improve the accuracy of predictive analytics.
- Real-time Insights:some text
- AI enables real-time analytics by processing data streams instantly, facilitating timely decision-making and proactive interventions.
- Anomaly Detection:some text
- AI algorithms can automatically identify anomalies or outliers in data, which is crucial for fraud detection, fault diagnosis, and risk management.
- Personalization and Recommendations:some text
- AI-driven analytics enable personalized marketing, product recommendations, and customer experience optimization based on individual preferences and behaviors.
- Automated Decision-making:some text
- AI can automate routine decisions based on predefined rules and learn from feedback to continuously improve decision-making processes.
- Interactive and Explainable Analytics:some text
- AI-powered analytics tools provide intuitive interfaces that allow users to interactively explore data and understand the underlying factors driving insights.
Applications of AI in Analytics
The application domains of AI-powered analytics are extensive and continually expanding across industries:
- Finance:some text
- AI-driven risk assessment, algorithmic trading, fraud detection, and personalized wealth management.
- Healthcare:some text
- Predictive diagnostics, personalized treatment plans, drug discovery, and patient outcome forecasting.
- Retail:some text
- Customer segmentation, demand forecasting, recommendation systems, and supply chain optimization.
- Manufacturing:some text
- Predictive maintenance, quality control, supply chain management, and production optimization.
- Telecommunications:some text
- Customer churn prediction, network optimization, and predictive maintenance of infrastructure.
- Marketing and Advertising:some text
- Targeted advertising, customer journey analysis, sentiment analysis, and campaign optimization.
Challenges and Considerations
Despite its transformative potential, integrating AI into analytics poses several challenges:
- Data Quality and Bias:some text
- AI models are sensitive to biases and inaccuracies in training data, leading to potential ethical and fairness concerns.
- Interpretability:some text
- Deep learning models often lack interpretability, making it challenging to understand and explain the rationale behind their decisions.
- Privacy and Security:some text
- Handling sensitive data raises privacy and security concerns, necessitating robust data governance and security measures.
- Skill Gap:some text
- There is a shortage of AI and analytics talent with the requisite skills to develop, deploy, and maintain AI-powered analytics systems.
Future Directions
The future of AI in analytics promises further advancements and innovations:
- Explainable AI:some text
- Efforts are underway to enhance the transparency and interpretability of AI models, enabling better trust and understanding.
- AI-Driven Automation:some text
- Automation of end-to-end analytics processes, from data preparation to insights generation, will become more prevalent.
- AI for Edge Analytics:some text
- AI algorithms will increasingly operate at the edge, enabling real-time insights and decision-making without reliance on centralized infrastructure.
- Ethical AI:some text
- Emphasis on developing ethical AI frameworks and guidelines to address biases, privacy concerns, and accountability in AI-driven analytics.