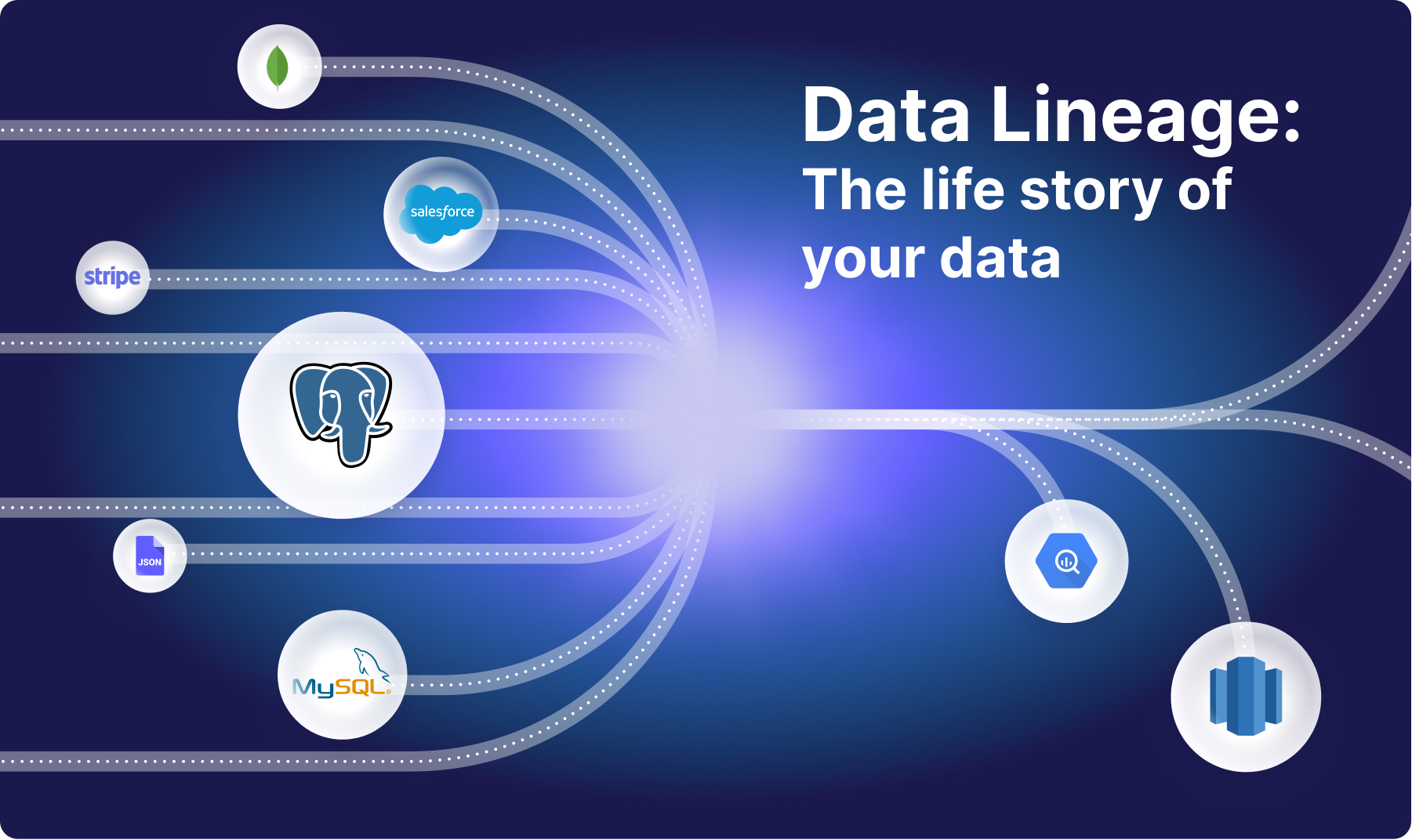
In today's data-driven landscape, the importance of understanding and managing data lineage cannot be overstated. Data lineage is not just a technical concept; it plays a pivotal role in ensuring data quality, governance, and compliance. This article delves deep into what the data lineage process is, why it matters, and how it impacts various aspects of data management.
What is Data Lineage?
Data lineage refers to the comprehensive view of the origin and movement of data from its source through various processes and systems, ending with data transformation at its destination or consumption point. It tracks the data's journey, providing transparency into how data is transformed, aggregated, and consumed across an organization.
Why Data Lineage Matters
Data lineage is crucial for several reasons:
- Data Quality: Understanding data lineage helps identify and rectify data quality issues by tracing back to their source.
- Compliance and Governance: It aids in regulatory compliance by ensuring data integrity, privacy, and security.
- Risk Management: It helps manage risks associated with data usage, transformations, and access.
- Operational Efficiency: Data lineage optimizes data management processes by streamlining data flows and enhancing transparency.
- Decision-Making: Enables better decision-making by providing insights into data origins and transformations.
Data Lineage Components
Data lineage encompasses the following key components:
- Data Sources: Origin points of data within the organization or external sources.
- Data Flows: Pathways through which data moves within systems and processes.
- Data Elements: Granular components of data, such as fields and attributes.
- Data Transformations: Changes applied to data during its journey, including aggregations, calculations, and mappings.
How Data Lineage Works
- Data Discovery: Identify relevant data sources and elements.
- Data Mapping: Establish relationships and dependencies between data entities.
- Tracking Data Movement: Monitor data flow across systems and processes.
- Metadata Management: Maintain metadata repositories for comprehensive lineage tracking.
- Visualization: Represent data lineage through visualizations for easier comprehension.
Types of Data Lineage
- Forward Data Lineage: Traces data from source to destination.
- Backward Data Lineage: Tracks data from destination back to source.
- Automated Data Lineage: Utilizes tools and technologies to automatically capture and visualize data flows.
- Manual Data Lineage: Relies on manual documentation and analysis to establish lineage.
Benefits of Data Lineage Tools
- Enhanced Data Governance: Facilitates adherence to data governance policies and standards.
- Improved Data Quality: Identifies and rectifies data quality issues promptly.
- Regulatory Compliance: Ensures compliance with data protection and privacy regulations.
- Impact Analysis: Enables quick identification of the impact of changes on downstream systems.
- Data Security: Helps in identifying and securing sensitive data elements.
Implementing Data Lineage
Implementing effective data lineage involves:
- Data Cataloging: Catalog all data assets and their associated metadata.
- Metadata Management: Establish a robust metadata repository.
- Integration with Data Management Processes: Integrate lineage tracking with data integration, transformation, and storage processes.
- Data Lineage Visualization: Use visual tools to represent data lineage for easier understanding.
List of Data Lineage Tools- In the dynamic landscape of data management, various specialized tools cater to the need for efficient data lineage tracking, visualization, and management. These tools are instrumental in enhancing data governance, ensuring data quality, and facilitating regulatory compliance. Below is a curated list of prominent data lineage tools that organizations can leverage to gain comprehensive visibility into their data flows.
- 1. Apache Atlas
- Apache Atlas is an open-source data governance and metadata framework that includes robust data lineage capabilities. It provides a scalable and extensible platform for capturing, managing, and visualizing data lineage across complex data ecosystems. Apache Atlas is widely adopted in big data environments due to its integration with Apache Hadoop and other ecosystem components.
- Key Features of Apache Atlas:
- Metadata Management: Apache Atlas offers a centralized metadata repository for storing and managing metadata related to data lineage, entities, and relationships.
- Graph Visualization: The tool provides graph-based visualizations of data lineage, allowing users to explore data flows and dependencies intuitively.
- Policy Enforcement: Apache Atlas supports policy-based governance, enabling organizations to enforce data governance policies across data assets.
- Integration: It integrates seamlessly with Apache Hadoop and other Apache ecosystem projects, making it ideal for managing lineage in big data environments.
- RESTful API: Apache Atlas provides a RESTful API for programmatic access to lineage data, enabling automation and integration with external systems.
- 2. Collibra Data Lineage
- Collibra Data Lineage is a part of the Collibra Data Intelligence Cloud, offering end-to-end data governance and lineage capabilities. It enables organizations to understand data origins, transformations, and usage patterns, empowering data-driven decision-making and compliance.
- Key Features of Collibra Data Lineage:
- Business Glossary Integration: Collibra integrates with business glossaries, enabling business users to understand and interpret data lineage within familiar contexts.
- Data Quality Monitoring: The tool includes data quality monitoring features along the lineage, providing insights into data health and integrity.
- Impact Analysis: Collibra supports impact analysis to assess the impact of changes on upstream and downstream systems, facilitating change management.
- Data Stewardship: Collibra facilitates data stewardship activities by providing visibility into data lineage and associated metadata.
- Collaboration: The platform enables collaboration among data stakeholders, fostering a data-driven culture within organizations.
- 3. Informatica Enterprise Data Catalog
- Informatica Enterprise Data Catalog offers robust data discovery, metadata management, and data lineage capabilities. It provides a unified view of data assets, their lineage, and relationships, enabling organizations to govern and manage data effectively.
- Key Features of Informatica Enterprise Data Catalog:
- Automated Lineage Discovery: The tool automatically discovers and captures data lineage across heterogeneous data sources and platforms.
- Metadata Management: Informatica Enterprise Data Catalog maintains a comprehensive metadata repository, ensuring accurate lineage tracking and governance.
- Data Profiling: The platform includes data profiling features to assess data quality and integrity along the lineage.
- Integration: Informatica integrates with various data integration and governance tools, enabling seamless lineage implementation within data ecosystems.
- Collaborative Features: Informatica supports collaboration among data stakeholders, fostering transparency and accountability in data management.
- 4. Alation Data Catalog
- Alation Data Catalog combines data cataloging, data governance, and data lineage capabilities to empower organizations with comprehensive data management solutions. It offers intuitive features for understanding data lineage, dependencies, and transformations.
- Key Features of Alation Data Catalog:
- Unified Data Catalog: Alation provides a unified view of data assets, including lineage information, data quality metrics, and usage patterns.
- Data Discovery: The platform enables easy discovery of relevant data assets and their lineage relationships.
- Collaborative Governance: Alation supports collaborative governance practices, facilitating data stewardship and accountability.
- Integration: The tool integrates with popular data platforms and tools, enabling seamless lineage tracking across diverse environments.
- Data Governance Dashboard: Alation offers interactive dashboards for monitoring data governance metrics, including lineage completeness and data quality.
- Data lineage tools are indispensable for organizations seeking to establish robust data governance practices and ensure data quality and compliance. Each tool listed above offers unique capabilities tailored to different organizational needs, data environments, and integration requirements. By leveraging these tools, organizations can gain actionable insights into their data lineage, improve decision-making, and drive operational efficiency in the data-driven era. When selecting a data lineage tool, organizations should consider factors such as scalability, ease of integration, and alignment with their specific data governance objectives.
FAQs: Answering Common Questions about What is Data Lineage
- What is the role of data lineage in data management processes? Data lineage ensures transparency and traceability in data processes, enhancing data quality and governance.
- How does data lineage help in regulatory compliance? By tracking data origins and transformations, data lineage aids in demonstrating compliance with data regulations.
- What are the challenges of implementing data lineage? Challenges include complex data ecosystems, disparate systems, and maintaining metadata consistency.
- How does data lineage impact data security? Data lineage identifies sensitive data elements and their movement, aiding in data security measures.
- What is the difference between forward and backward data lineage? Forward lineage tracks data from source to destination, while backward lineage traces data from destination back to source.
- How does data lineage contribute to data quality improvement? By identifying data origins and transformations, lineage helps pinpoint and rectify data quality issues.
- What role do data engineers play in managing data lineage? Data engineers are instrumental in implementing and maintaining data lineage processes and tools.
- How can data lineage support data migrations? Data lineage ensures a smooth migration process by mapping dependencies and impacts of data movements.
- What is the significance of automated data lineage solutions? Automated solutions reduce manual effort, provide real-time insights, and ensure accuracy in data lineage tracking.
- How does data lineage impact business users? Business users benefit from data lineage by gaining insights into data origins, quality, and impact on business processes.
- What is the relationship between data lineage and data governance policies? Data lineage supports data governance by ensuring compliance with policies and standards through transparent data tracking.
- How does data lineage help in understanding data transformations? Data lineage reveals how data is transformed through various stages, aiding in understanding data transformations.
- What types of data assets are tracked in data lineage? Data lineage tracks all types of data assets, including structured, unstructured, and semi-structured data.
- How can organizations perform impact analysis using data lineage? Impact analysis involves tracing the impact of changes on downstream systems, which is facilitated by data lineage.
- What is the role of data lineage in big data environments? Data lineage is crucial in big data environments for understanding complex data flows and ensuring data quality.
- How does data lineage assist in data tagging and classification? By tracking data origins and attributes, lineage facilitates accurate tagging and classification of data.
- What is the importance of metadata management in data lineage? Metadata management ensures comprehensive and accurate lineage tracking by maintaining metadata repositories.
- How does data lineage assist in managing data lifecycle? Data lineage provides insights into the data lifecycle by tracking data from creation to deletion or archiving.
- What are the best practices for implementing data lineage in modern data environments? Best practices include automated lineage capture, metadata standardization, and integration with data governance frameworks.
- How can organizations leverage data lineage for risk management? Data lineage helps identify and mitigate risks associated with data usage, security, and compliance.
Conclusion
In conclusion, data lineage is a critical aspect of modern data management, offering transparency, traceability, and insights into data processes. Organizations that prioritize modern data lineage will benefit from improved data quality, governance, and compliance, ultimately driving better decision-making and operational efficiency in a data-centric world.